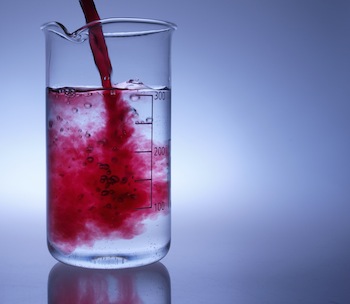
Yeah ... I'm going to take a stab and say that has never happened. Why? According a report by Econsultancy, only 28% of marketers are satisfied with their conversion rates. It's more than likely that your team falls into that unsatisfied bucket.
One of the best ways to get out of that conversion rut is to embrace your mathematical side and run an A/B test. It's a kind of experiment that shows two different versions of one piece of content (such as a landing page, email, or call-to-action) to two similarly sized audiences to see which one performs better.
(Bonus: You can use A/B tests in a ton of different ways in your marketing, and they're relatively simple to execute.)
If you're one of those marketers who isn't satisfied with your conversion rates, keep reading. I'll walk you through each step of designing, implementing, and measuring an A/B test. You can use these steps for anything in your marketing -- we're going to explain the process using calls-to-actions (CTAs) for our example.
Want to follow along to help get your own A/B test up and running by the end of this post?Download our free call-to-action PowerPoint template to cut your design time in half.
Ready to run an A/B test? Here's how you do it.
1) Decide what you're going to test.
The great thing about A/B tests is that you have two pieces of content to test -- but you can test both large and small elements in your marketing. You can test something as small as the color of a CTA to something as big as an entirely redesigned page.
The only thing that you need to remember as you add more differences between the two pieces of content is that you can only attribute the results to each piece of content you're testing as a whole -- not individual differences. This means that if you're testing two versions of one landing page against each other, and you've changed the CTA copy, the form length, the image you've added, and the headline copy on one of the landing pages, you cannot attribute that landing page's success to the form itself. You'd have to attribute success to all four of those elements.
Need ideas for your own A/B test? Check out this article for some small, quick wins and this post from KISSmetrics for advice on running larger A/B tests. If you're trying to fix your visitor-to-lead conversion rate, I'd recommend trying some landing page, email, or call-to-action A/B test.
For the purpose of this article, we've decided to run an A/B on our CTAs to what changing the color of the button does.
2) Figure out the goal of your test and decide how to measure it.
To run a successful A/B test, we can't just hit the ground running after reading the last sentence of the previous step. You've got to think deeper about what you want to find out through the A/B test. Do you want to measure how the color of the CTA affects how many people click on it? That's the most straightforward test you could do. But you could also test to see if the color affects how many people click more than once on the CTA.
In our example A/B test, we want to send lots of people to the landing page, so we're going to use the number of clicks on the CTA as our indicator of success.
3) Set your control and treatment.
Ignore the jargon -- the control and treatment of your A/B test are quite simple. The control is simply the "Version A" of your test -- it's what you normally use as your landing page, email, call-to-action, headline, etc. The treatment is the "Version B" of your test -- it's the version that has the changes you're trying to test.
In our example, the control (Version A) would be a dark grey -- the color of most of our blog CTAs. It's the status quo, the norm. The treatment (Version B) would be something different -- let's say a bright blue.
4) Create your A/B test and release it into the wild.
Once you've design how your experiment is going to work, get on with making it! First, actually design and create the content for your control and treatments: in our case, the grey CTA and the blue CTA below. Notice how the only thing different between the two is the color -- the copy and images used are the same on both. That way, we can actually test whether color affected the number of clicks.
Variation A:
Variation B:
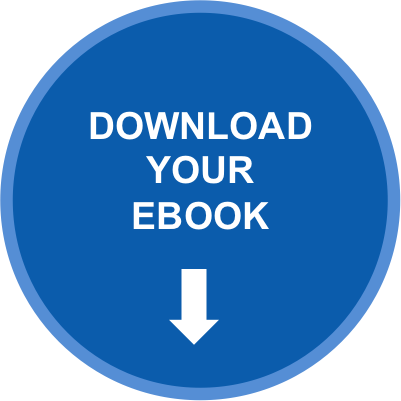
Then, you'll have to set up the A/B test in your marketing software. Each tool is different, and often, the A/B testing steps are different for each type of content you're going to test. If you're a HubSpot Enterprise customer who is following along with our example, you can use these instructions. If you're a HubSpot Enterprise customer running a different A/B test, here are other resources to help you out.
5) Promote your test -- but maybe not to everyone.
If you want to have your test mean anything at all -- in other words, be statistically significant, which we'll go over in the next bullet point -- you'll have to promote the heck out of your content. Send your email to a large enough list, promote your landing page across social, or even throw some PPC behind the blog post link to get enough people to see your test.
Keep in mind that if you're running an A/B test for a specific audience, you need to keep your promotions tailored to only that audience. For example, let's say that you're curious if Twitter followers will like something on a landing page, you wouldn't want to promote the A/B test content anywhere other than Twitter. Not on Facebook, not through email -- just through Twitter.
In our example, we're just looking at CTA conversions, so we'd reeeeeeally promote the blog post to get whoever is interested to the page.
6) Gather data until it's significant.
Now comes the waiting game. Keep promoting your test until it's statistically significant -- one way of saying when the results of your tests are determined to be most likely not due to chance alone. If you're really into math and statistics, you can calculate significance yourself following the steps here. Otherwise, you can use this handy dandy tool. Once you've hit significance, you can see if the treatment is more effective than the control.
But what happens if you never hit significance? Wait a few more days. Sometimes, it can take up to 30 days to get enough traffic to your content to get significant results. That being said, if it's been a month and you've sent a lot of traffic to your test, but you haven’t seen a statistically significant result, then your test probably wouldn’t make a big impact on conversions. Don’t be afraid to move on to another experiment.
7) Investigate your entire marketing funnel.
Okay, so now you know if your experiment worked or not for the metrics you originally set. Awesome! But you can't stop there.
Even though I told you to only focus on one metric before, this is the one time in the test where you can look outside the test's original purpose to see if it's had effects on any other part of your marketing funnel.
While it might be silly to think that changing the color on your CTA could impact anything other than the number of clickthroughs, it can happen. If you have closed-loop analytics, you can track to see if people who clicked on that CTA actually become customers. Maybe the people who click on blue CTAs become customers faster.
That's probably a ridiculous claim, but you see what I mean. By looking at other parts of your marketing process, you may discover that an A/B test has consequences you didn't anticipate. And if those consequences are good, you can lean harder into them. Otherwise, you might want to rethink whether you should make that change.
Just remember that your A/B test can have larger implications than just the test metrics themselves.
8) Iterate on your findings.
Now, you've gathered your data -- significant or not -- and checked to see if your A/B test had any other unintended consequences. You're done!
Just kidding. You've finished your first A/B test -- and that's cause for celebration -- but there's so much more you can test. In the CTA example, you can try placing the CTA elsewhere on the page or see if switching up copy can affect how many people click through.
Or maybe you just don't trust the results of the A/B test you just ran. Maybe you ran it during a holiday and got a ton of traffic to your site -- but that's not indicative of how your audience normally behaves. Run the A/B test again -- except make sure you're not doing it during a holiday.
If you're always testing, you can make great strides in your conversion rates -- and hey, maybe even make your boss happy enough to be "satisfied" with his or her team's conversion rates. Good luck!